Activating Data Mesh 💡
Case study on scaling data collaboration, governance, quality, and ownership across 60 data teams – bringing data mesh to life.
Founded in 1982, and since growing to $5 billion in annual revenue and nearly 14,000 employees, Autodesk affected seismic change for architects, engineers, and designers when it introduced Computer-aided Design. In the decades since, the company has grown into a leading, cloud-first technology company, offering dozens of products and services, supporting diverse users from Media & Entertainment to Industrial Bioscience.
In this edition of the newsletter, read highlights from our conversation with Mark Kidwell, Chief Data Architect, Data Platforms and Services at Autodesk on how their data team overcame the challenge of scaling data collaboration and governance across 60 data teams with distinct ownership models and built the data mesh that was right for them.
You can read the complete story here →
“A lot of folks may know Autodesk as the AutoCAD company, or might have used it in the past for design in architecture engineering, or construction. It’s moved way beyond that. Those are our roots, but we now provide software, and empower innovators with all sorts of design technology, in addition to product design and manufacturing.” – Mark Kidwell, Chief Data Architect, Data Platforms and Services at Autodesk.
Underpinning this transformation, from AutoCAD pioneer to Nasdaq 100 technology leader, is data-driven decision-making, powered by a visionary data team, and modern data technology like Atlan and Snowflake.
Autodesk’s Analytics Data Platform
While the Analytics Data Platform Group’s mission of enabling analytics is simply summarized, the team’s responsibilities are vast and complex. Their services include maintaining a number of core engines, data warehouses, data lakes and metastores. They provide ELT services, as well as ingestion, transformation, publishing, and orchestration tools to manage workloads, and analytics services like BI layers, dashboarding, and notebooks. And to coordinate these services, they drive a set of common tooling that enables data governance, discovery, security monitoring, and DataOps processes like pushing pipelines to production.
“We power both BI Analytics as well as a ton of ad-hoc analytics,” Mark shared. “We’re also used more for process reconciliation, an integration layer for a lot of data, and we can also power a single view of customer use cases. We’re enabling teams to push data to downstream systems after building data products on our platform. And finally, everyone’s favorite topic, AI and ML are a feature of the platform, as well.”
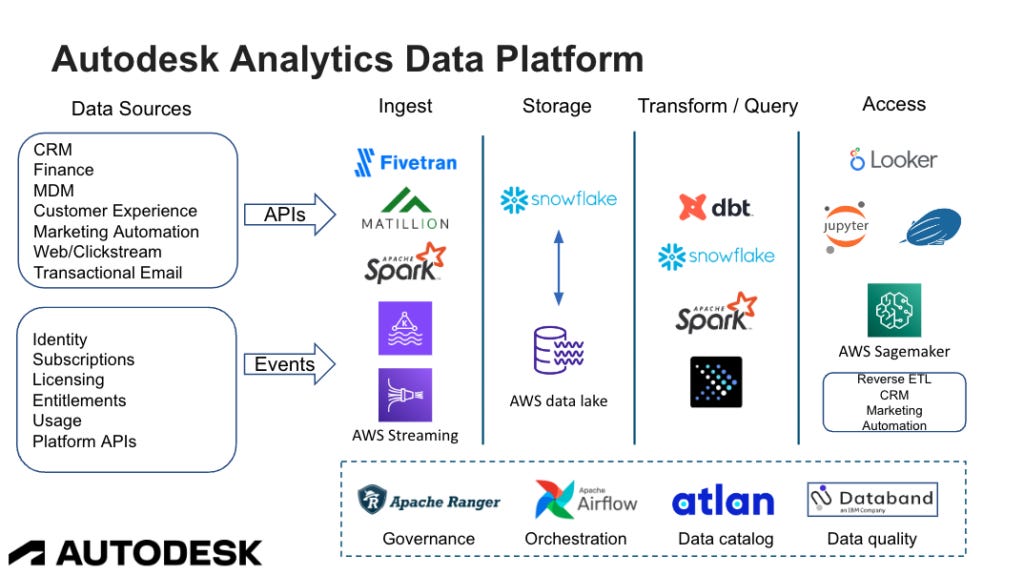
“We use a combination of a data lake and a data warehouse. Our data warehouse is Snowflake, the data lake is AWS, and of course, all the technology sits on top of the lake and warehouse to run transformations, queries, and analytics,” Mark shared. “We’ve adopted a lot of the tools and technologies that are part of the Modern Data Stack, but we have a lot of use cases that require us to maintain the data lake for our high volume and high velocity data sets that generate events.”
Rounding out their modern data stack are a series of technologies they refer to as their access layer, like Looker, PowerBI, Notebooks, and AWS Sagemaker, as well as Reverse ETL tools to push data back into other systems.
Bringing Data Mesh from Concept to Reality
Over the course of their previous work, the Analytics Data Platform team had already made progress against Zhamak Dehghani’s four core pillars of Data Mesh, but in order to further translate these concepts into a strategy that met their needs, the team began a gap analysis to see where they could improve. Moving pillar-by-pillar, Mark’s team began mapping potential improvements to their two key audiences: Producers and Consumers.
Decentralized Domain Ownership
The first pillar, Decentralized Domain Ownership and Architecture, ensures that the technology and teams responsible for creating and consuming data can scale as sources, use cases, and consumption of data increases.
“We had a long history of supporting data domains and different teams working on the platform, owning those domains. They were acting relatively independently, and perhaps too independently,” Mark shared. “A real challenge for us was finding data that these domain owners had brought into the system. And if you were a consumer with an analytics question, a common complaint was that they had no idea an asset was there, or how to find it.”
Data as a Product
The second pillar, Data as a Product, ensures data consumers can locate and understand data in a secure, compliant manner across multiple domains.
“A consistent definition of a data product meant defining what teams are expected to do in terms of defining product requirements, or what they are expected to do in terms of meeting data contracts and SLAs,” Mark explained. “We would have to move from teams that were simply ingesting data, and toward teams that were thoughtfully publishing data on the platform and thinking about what it meant to their consumers to have these data.”
Self-service Architecture
The third pillar, Self-service Architecture, ensures that the complexity of building and running interoperable data products is abstracted from domain teams, simplifying the creation and consumption of data.
“There are so many ways to define self-service. You could say we were self-service when we had Spark and people could write code,” Mark explained. “We were definitely better at self-service once we adopted no-code and low-code tools, but even if you used all those tools directly, there was no guarantee you would get the same results. Different teams might use them, and it results in a completely different data product. So we wanted to make sure that not only were we using self-service at the tool level, but we were providing frameworks or other reusable components.”
Federated Computational Governance
The fourth and final pillar, Federated Computational Governance, ensures the Data Mesh is interoperable and behaving as an ecosystem, maintaining high standards for quality and security, and that users can derive value from aggregated and correlated data products.
At the time, Autodesk was early in their data governance journey, making it difficult for the platform team to understand how their platform was used, for publishers to understand who consumed their products, and for consumers to get access to products.
“We couldn’t move forward with a lot of other things we wanted to do if we didn’t have a stronger governance footprint. This led to a series of workstreams for us, and a more crisp definition of who the different personas and roles using the platform were.”
Defining Workstreams to Support Publishers and Consumers
The Autodesk team began by formally defining the roles of publishers, consumers, and the platform team, then defined workstreams that improved discrete parts of the Analytics Data platform, organized by the persona they would benefit. Top priority was given to workstreams that would benefit publishers, including platform-wide standards, and the processes and tools necessary to easily ingest and publish secure, compliant data.
Consumer workstreams focused on trust, ensuring that sensitive data could be shared on the platform, and that they had the tools they needed to discover and apply data. Finally, Data Platform workstreams ensured that Mark’s team could enforce quality standards, and understand data product consumption and its associated costs.
To this point, the Analytics Data Platform team was responsible for data engineering and defining product requirements, and knew the tools, data, and consumers for the data products that they built. But to drive trusted data at scale, each publishing team would need to learn these skills, as well.
“We don’t scale this by scaling up the core team. We had to enable other teams to do all these things,” Mark explained. “It meant that instead of [only] the core platform team knowing and using the tools to deliver products directly, we had to enable publisher teams to have their own data product owners and their own data engineers.”
Each of Autodesk’s publishing teams would need to define a Product Owner and Data Engineers. Product Owners would ensure that consumer requirements were understood, and Data Engineers would have the necessary expertise to use platform tools, and ensure high technical standards. Repeating the process across one publishing team after another, the Analytics Data Platform team would provide the tooling, standards, and enablement necessary for each publishing team to be successful.
Just two years later, Autodesk has successfully ingested dozens of data sources, and has built numerous data products, all delivered by either individual teams, or combinations of teams building composite data sources from multiple domains like Enterprise and Product Usage data.
Since we started the self-service initiative, we’ve had a total of 45 use cases that have gone through since 2021. It’s not something that we could have done if we just had one core ingestion team; one core data product team.”
Mark Kidwell, Chief Data Architect, Data Platforms and Services
Read more about how the Autodesk team is bringing data mesh to life with Atlan →
🗓 Atlan Activate: Bringing Data Mesh to Life
Every tool is trying to force-fit features to data mesh concepts. But that’s not the experience that humans of data deserve.
Join us for the reveal of the first-ever native data mesh experience in a data catalog. See all-new data products, domains, and contracts come to life as first-class citizens in Atlan. Sign up here →
P.S. Liked reading this edition of the newsletter? I would love it if you could take a moment and share it with your friends on social! If someone shared this with you, subscribe to upcoming issues here.